Artificial Intelligence has been subtly altering our world for years, laying the groundwork for advancements across various fields. One such area is AIOps, a groundbreaking application of AI designed to optimize and automate IT processes.
Whether you are a professional looking to streamline your workflow or a decision-maker evaluating cutting-edge technologies, AIOps offers a compelling proposition. It can automate complex processes, boost efficiency, and resolve issues with unparalleled speed and precision.
This article explains what AIOps is and how it is reshaping IT operations.
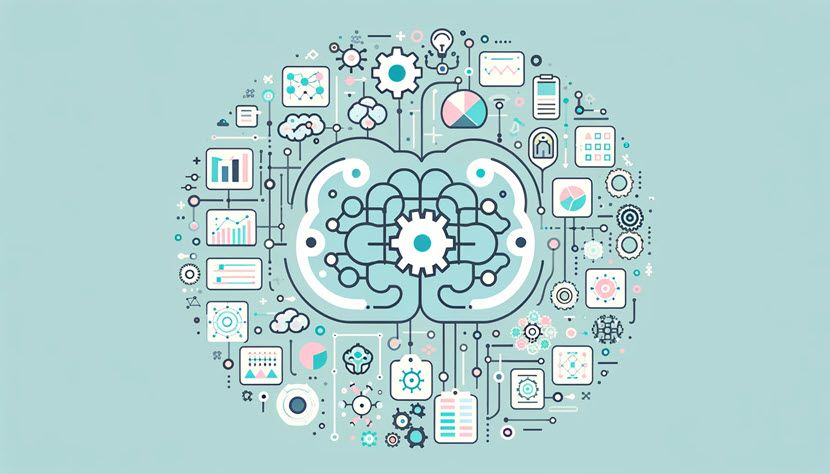
What Is AIOps?
AIOps, short for artificial intelligence for IT Operations, is a framework that combines big data and machine learning to automate and enhance IT operations. It leverages advanced algorithms to monitor and analyze data from every corner of an IT environment, providing DevOps and ITOps teams with actionable insights and automation capabilities. AIOps does not replace human involvement but rather fills operational gaps.
Standing at the junction of all monitoring, log management, and orchestration tools, AIOps processes and integrates information across the entire IT infrastructure. This integration creates a synchronized, 360-degree view of operations, making it easier to track and manage. Using specialized algorithms focused on specific tasks, AIOps platforms filter alerts from noisy event streams, identify correlations, and auto-resolve recurring problems using historical data. The cumulative effect boosts system stability and performance, preventing issues from impairing critical operations.
AIOps solutions can be either custom-built or out-of-the-box managed services. Out-of-the-box solutions offer quick and reliable deployment with vendor support while building your own provides maximum customization and control. A hybrid approach combines the best of both worlds. The choice depends on your organization’s needs, resources, and expertise.
What Is the Difference Between AI and AIOps?
AI is a broad field that includes various technologies and methodologies for creating systems capable of performing tasks that typically require human intelligence. The field of AI includes machine learning, natural language processing, deep learning, computer vision, neural networks, and more.
AIOps is a specialized application of AI designed specifically for IT operations. It uses machine learning to enhance and automate IT operations processes, including monitoring, event correlation, anomaly detection, and incident management.
Discover the leading AI processors that can accelerate your projects.
Types of AIOps
AIOps platforms are categorized based on their functionality, deployment models, and the specific problems they solve.
Below is a comprehensive classification of the types of AIOps.
Functional Types of AIOps
AIOps solutions address various aspects of IT operations.
- Event correlation and analysis. These tools gather data from various sources, correlating events and identifying patterns. They can pinpoint the root cause of an outage, reduce alert noise, and give you a clearer picture of the incident.
- Anomaly detection. Anomaly detection systems continuously monitor data, identifying deviations and acting as early warning systems. For example, network monitoring systems flag unusual spikes, indicating data breaches or performance problems.
- Predictive analytics. Predictive AIOps tools use machine learning to forecast incidents or capacity needs. For example, a predictive analytics tool can predict server failures based on historical data and current conditions and recommend preventive actions.
- Automated root cause analysis. These solutions automate incident diagnostics by analyzing data from multiple sources to pinpoint the underlying issue quickly, reducing the time to resolution.
- Process automation. AIOps platforms automate routine tasks to reduce manual effort and improve operational efficiency. For example, they can automatically patch vulnerable systems when they are identified.
- Capacity optimization. Capacity optimization tools use AI to analyze resource utilization and optimize the allocation of IT resources. For example, these systems can dynamically adjust virtual machine resources based on demand to maintain performance while minimizing costs.
- Log and metric management. These tools collect, store, and analyze logs and metrics. They provide insights into system performance and help identify trends and anomalies.
- Service impact analysis. These AI tools assess the impact of incidents on IT services and business processes. For example, they can analyze the impact of a network outage on different business applications and prioritize restoration efforts accordingly.
- Security incident response. These solutions enhance cybersecurity by automating detection and response to cyber incidents. For example, endpoint security systems automatically detect and isolate compromised endpoints based on security alerts and behavior analysis.
Deployment Models
You can deploy an AIOps solution in three models, each offering different advantages:
- On-premise. In this model, you deploy the solution within the organization’s data centers. This option provides more control over data and infrastructure.
- Cloud-based. Hosting the solution in the cloud offers scalability, flexibility, and reduced infrastructure management overhead.
- Hybrid. This model combines on-premises and cloud-based deployments to leverage the benefits of both.
Scope and Applicability
AIOps solutions are also classified based on their scope and applicability within an organization's IT environment.
Domain-Centric AIOps
Domain-centric AIOps solutions are tailored to specific areas or domains within IT operations. They address specialized needs and provide deep insights and automation within a particular scope. Here are examples of the areas domain-centric AIOps focus on:
- Networking. Tools that monitor and optimize network performance, identify bottlenecks, and automate network management tasks.
- Application performance. Solutions that track application metrics, detect performance issues, and provide automated incident response for application-level problems.
- Cloud computing. Platforms that manage cloud resources, ensure compliance, and optimize cloud infrastructure usage.
- Suggest solutions. AIOps tools recommend the most appropriate action based on historical data and pre-defined troubleshooting protocols.
- Self-healing actions. AIOps tools automatically trigger pre-defined remediation actions to resolve issues without human intervention, improving response times.
Domain-Agnostic AIOps
Domain-agnostic AIOps solutions are versatile and can be applied across various domains and IT environments. They are designed to scale predictive analytics and AI automation beyond specific operational areas, providing a more holistic view of IT operations. IT teams can use domain-agnostic AIOps to integrate data from multiple sources, correlate events across different systems, and derive comprehensive business insights.
Here are examples of domain-agnostic AIOps:
- Cross-domain event correlation. Tools that aggregate and correlate events from diverse IT environments, such as servers, applications, networks, and security systems.
- Predictive analytics across boundaries. Solutions that provide predictive maintenance and performance optimization across the entire IT infrastructure, regardless of the specific domain.
CPUs struggle with the demanding computational needs of training AIOps platforms. GPUs offer a dramatic performance leap, significantly accelerating the training process. Our in-depth exploration of GPUs for deep learning explains how these specialized processors unlock the full potential of your AIOps, enabling faster training times and optimal performance.
AIOps Tools
Below is an overview of the essential AIOps tools and their features, pros, cons, and pricing.
Datadog
Datadog is a popular AIOps platform offering real-time monitoring across various IT components, including servers, databases, applications, and cloud services. Its AI capabilities pinpoint anomalies and streamline troubleshooting.
Pros:
- Real-time monitoring. Provides comprehensive visibility into your IT infrastructure.
- AI-powered insights. Uses machine learning to identify issues and automate tasks.
- Cloud-native architecture. Scales easily to accommodate growing IT needs.
Cons:
- Limited free tier. Free plan offers basic functionalities with limited data ingestion.
- Complex for beginners. The feature-rich interface has a learning curve.
Pricing:
- Free tier for small setups (up to 5 hosts) with basic features.
- Paid tiers offer extended data retention, more integrations, security features, and per-host pricing. Custom metrics have a free tier quota and a pay-as-you-go option.
Splunk
Splunk is a versatile security information and event management (SIEM) platform that collects, analyzes, and visualizes machine data from diverse sources. Its AIOps features encompass anomaly detection, root cause analysis, and automated remediation.
Pros:
- Platform flexibility. Integrates with a wide range of IT systems and data sources.
- Strong data analytics capabilities. Offers powerful tools for data exploration and visualization.
- Scalability. Manages large datasets efficiently.
Cons:
- Steep learning curve. Complex to set up and customize.
- Pricing structure. Expensive for large deployments.
Pricing:
- Free version available with limited features.
- Sixty-day free trial.
- Splunk offers various pricing tiers based on data usage and features (custom quote).
LogicMonitor
LogicMonitor provides a comprehensive IT infrastructure monitoring solution that incorporates AIOps functionalities like real-time anomaly detection, root cause analysis, and automated workflows.
Pros:
- User-friendly interface. Easy to navigate and understand, even for beginners.
- Pre-built integrations. Supports a wide range of IT tools and platforms out of the box.
- Cost-effective. Competitive pricing compared to other options.
Cons:
- Limited customization. Customization options might be less extensive compared to some competitors.
- Focus on infrastructure monitoring. Not ideal for application-centric needs.
Pricing:
- Free trial available.
- Paid plans start around $22 per resource/month (e.g., servers, VMs) with volume discounts.
- Log intelligence pricing varies from $4 to $14 per GB/month based on data retention needs.
Dynatrace
Dynatrace offers application performance management (APM) with built-in AIOps functionalities. It leverages AI to pinpoint performance issues, automate root cause analysis, and recommend remediation actions.
Pros:
- Application-centric approach. Provides deep insights into application health and performance.
- Automatic root cause analysis. Reduces troubleshooting time by pinpointing problem sources quickly.
- Proactive performance optimization. Helps prevent performance issues before they impact users.
Cons:
- Pricing. Expensive for large deployments.
- Limited infrastructure monitoring. Primarily focused on application performance.
Pricing:
- Full-stack monitoring starts at $0.08 per hour for an 8 GiB host.
- Infrastructure monitoring starts at $0.04 per hour for any size host.
- Real user monitoring starts at $0.00225 per user session.
New Relic
New Relic offers a full-stack observability platform with AIOps features such as anomaly detection, incident alerting, and even automated incident resolution.
Pros:
- Full-stack visibility. Monitors infrastructure, applications, and user experience in a unified platform.
- Automated incident resolution. Reduces manual intervention and streamlines problem-solving.
- Focus on developer experience. Provides tools and integrations for developers to monitor and troubleshoot applications.
Cons:
- Complexity. The feature-rich platform might require some learning effort.
- Pricing. Can be expensive for large deployments with complex needs.
Pricing:
- Free tier available with 100 GB data/month and one full user.
- Pro plans charge extra for data over 100 GB at $0.30 per GB.
- Prices for additional users depend on the plan (Standard, Pro, Enterprise).
Looking for New Relic alternatives? Our article explores the best monitoring tools that offer strong features without breaking the bank.
How Does AIOps Work?
AIOps uses machine learning and data analytics to automate and optimize IT processes. Here is a closer look:
Intelligent Alert Management
Traditional IT monitoring often generates a flood of alerts, leading to "alert fatigue” and missed critical issues. AIOps addresses this challenge by:
- Correlating events. AIOps tools identify relationships between seemingly unrelated events and pinpoint the root cause.
- Prioritizing alerts. AIOps tools distinguish critical issues from less urgent ones, reducing alert fatigue and allowing IT teams to focus on high-priority incidents.
- Suppressing redundant alerts. AIOps tools eliminate repetitive or irrelevant alerts and streamline alert management.
Improved Situational Awareness
AIOps aggregates data across the entire IT infrastructure, including network devices, applications, servers, and cloud platforms. This holistic view enables IT teams to:
- Identify performance bottlenecks. AIOps tools proactively identify areas of potential performance degradation before they impact users or applications.
- Predict and prevent outages. AIOps tools predict potential outages and take preventive measures by analyzing historical trends and system behavior.
- Facilitate root cause analysis. When an incident occurs, AIOps tools analyze historical data and real-time events to pinpoint the root cause more quickly and accurately.
Automated Remediation
AIOps tools automate remediation actions for recurring issues. By learning from past incidents and identifying patterns, they can:
Proactive Performance Monitoring
AIOps utilizes AI-based analytics to proactively monitor application performance metrics such as resource utilization, bandwidth, CPU, memory, and response times. This allows for:
- Early detection of issues. Early identification of potential performance bottlenecks before they significantly impact user experience.
- Capacity planning and optimization. AIOps tools optimize resource allocation and predict future capacity needs based on historical trends and usage patterns.
Advanced Analytics and Cohort Analysis
AIOps excels at analyzing massive datasets from various IT tools and systems. This capability allows for:
- User behavior analysis. AIOps tools conduct in-depth analysis of specific user groups (cohorts) within the system, providing valuable insights into user behavior and application usage patterns.
- Security threat detection. AIOps tools analyze network traffic patterns and system logs to identify potential security threats and anomalies.
- Predictive maintenance. AIOps tools analyze sensor data from equipment to predict potential failures and schedule preventative maintenance, reducing downtime and costs.
AIOps Benefits
Here are the benefits of implementing AIOps:
Improved Efficiency
AIOps automates routine tasks like monitoring and log analysis, freeing staff from repetitive processes. The extra time allows IT teams to focus on strategic initiatives like cloud migration or security improvement projects.
Reduced Downtime
By proactively identifying potential problems, AIOps helps prevent outages before they occur. The reduction in downtime translates to improved service availability for end-users and minimized financial losses for the organization.
Enhanced Decision-Making
AIOps can identify trends and patterns that may not be obvious to humans. Your organization can use these insights to make more informed decisions about resource allocation, capacity planning, and overall IT strategy.
Stronger Security
AIOps analyzes data from firewalls, intrusion detection systems, and other tools to quickly detect and respond to threats. Additionally, machine learning algorithms can identify anomalies in network traffic or system behavior that may indicate a security breach.
What Are the Challenges of AIOps?
Implementing AIOps requires overcoming several challenges.
Pre-Implementation Concerns
Before deploying AIOps, you must address the following foundational elements.
- Alert definition. It is critical to establish clear definitions for alerts and their corresponding workflows, particularly in dynamic cloud environments with transient resources. A purely AI-driven approach, without proper definition, will not deliver optimal results.
- AI implementation challenges. Setting up and maintaining effective solutions within AIOps is complex. These solutions require significant data volumes, domain expertise for training, and vendor support, which often translates to substantial costs.
Data Management
AIOps is heavily reliant on data for training and operation. However, managing this data presents its own set of challenges:
- Data volume, variety, and velocity. The sheer volume, diverse formats, and real-time nature (velocity) of data an AIOps platform requires make management and integration a significant hurdle.
- Data quality concerns. Inaccurate or incomplete data leads to unreliable insights and hinders the effectiveness of AIOps. Ensuring data quality is essential for successful implementation.
Post-Implementation Challenges
Implementing an AIOps solution is only half the battle – integration and effective management are just as vital.
- Integration complexity. Integrating AIOps with existing IT infrastructure, which may include a mix of modern and legacy systems, requires careful planning and execution.
- Skilled personnel requirements. While AIOps automates tasks, it still demands skilled personnel to interpret results, manage the platform, and address issues that fall outside the scope of its capabilities.
- Security considerations. AIOps systems must be designed and implemented with robust security to protect sensitive data.
IT Operations with Artificial Intelligence
While many elements of AIOps have existed under different names, the convergence of machine learning and big data analytics has undoubtedly led to significant advancement in this field. AIOps is not simply a rebranding of existing tools—its potential to automate tasks, identify patterns, and predict issues is truly transformative for IT operations.
However, a cautious approach is essential. IT environments are complex, and implementing innovative technologies requires careful planning and execution. AIOps should be viewed as a tool to augment existing workflows, not a complete replacement. A measured approach ensures that integrations are smooth and minimize disruption. By prioritizing stability and taking a step-by-step approach, you can leverage the power of AIOps to optimize performance and proactively address potential issues without hindering overall efficiency.